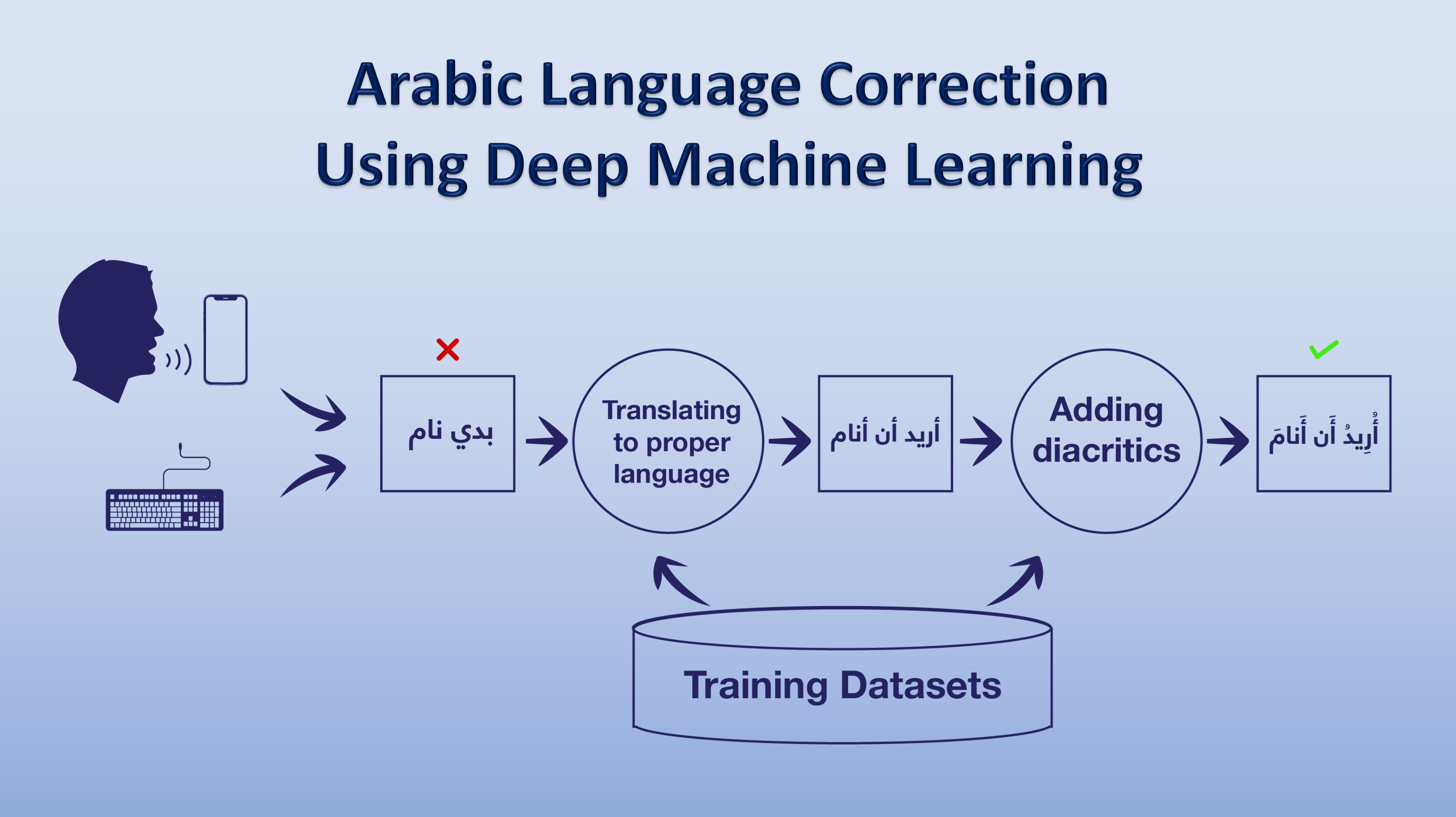
Research Overview
A dedicated team of researchers from the University of Jordan has embarked on an ambitious journey on a project titled "Developing Applications to Correct Jordanian Spoken Arabic to Proper Language Using Machine Learning Techniques".
Many Arabic speakers face challenges in expressing themselves in formal Arabic, leading to written texts riddled with errors or a resort to informal dialects. The research team, led by the Principal Investigator Prof. Gheith Abandah, seeks to address this issue by developing smartphone and computer applications that allow users to correct Arabic texts, converting them from informal dialects or error-laden texts into well-structured, diacritized Arabic.
Objectives
The five main project objectives are:
- Develop user-friendly applications accessible on smartphones and computers for correcting Arabic texts.
- Enhance communication by eliminating linguistic errors and confusion.
- Improve the quality of Arabic content on the internet.
- Contribute to the advancement of computer support for the Arabic language in Jordan and the wider Arab world.
- Provide datasets for Arabic language research and develop algorithms using machine learning techniques.
Research Methodology
The research focuses on deep machine learning techniques, necessitating the collection of diverse Arabic sentences from Jordanian users with linguistic errors or in the local informal dialect, and annotating these sentences with their respective proper Arabic translations. The team aims to answer the central research question: What deep machine learning models and training techniques are suitable for efficiently correcting Arabic language mistakes and translating Jordanian dialect text to formal Arabic?
To overcome the challenge of acquiring many samples, the team plans to use synthetic samples and employ transfer learning methods. These approaches will facilitate the development of machine learning solutions to translate input text into valid and diacritized Arabic. The project has obtained high-performance workstations equipped with advanced hardware computation accelerators to expedite the training of deep learning experiments.
This research extends upon prior achievements and advancements in the realm of addressing challenges in the Arabic language through the application of deep learning techniques. These include the recognition of handwritten Arabic text [1], the automatic diacritization of Arabic texts [2], the classification and diacritization of Arabic poetry [3, 4], the correction of Arabic spelling mistakes [5], and the creation of Arabic chatbots [6].
Expected Impact
The outcomes of this project are anticipated to revolutionize Arabic language correction, enhance communication, and contribute valuable datasets and algorithms to the broader field of natural language processing. By providing accessible applications to users in Jordan, the project aims to empower individuals to communicate effectively in formal Arabic, thereby positively influencing linguistic skills and content quality on the internet.
As the project unfolds, the University of Jordan's research team anticipates making substantial contributions to the advancement of computer support for the Arabic language in Jordan and the Arab world at large.
Project Team
The interdisciplinary team, comprising members from the Computer Engineering Department and the Department of Arabic Language and Literature, is led by Prof. Gheith Abandah. The team includes experts like Prof. Iyad Jafar, Dr. Mohammad Abdel-Majeed, Dr. Yousef Hamdan, Dr. Ashraf Suyyagh, Eng. Asma Abdel-Kareem, three Data Analytics research assistants, and four Arabic Language research assistants.
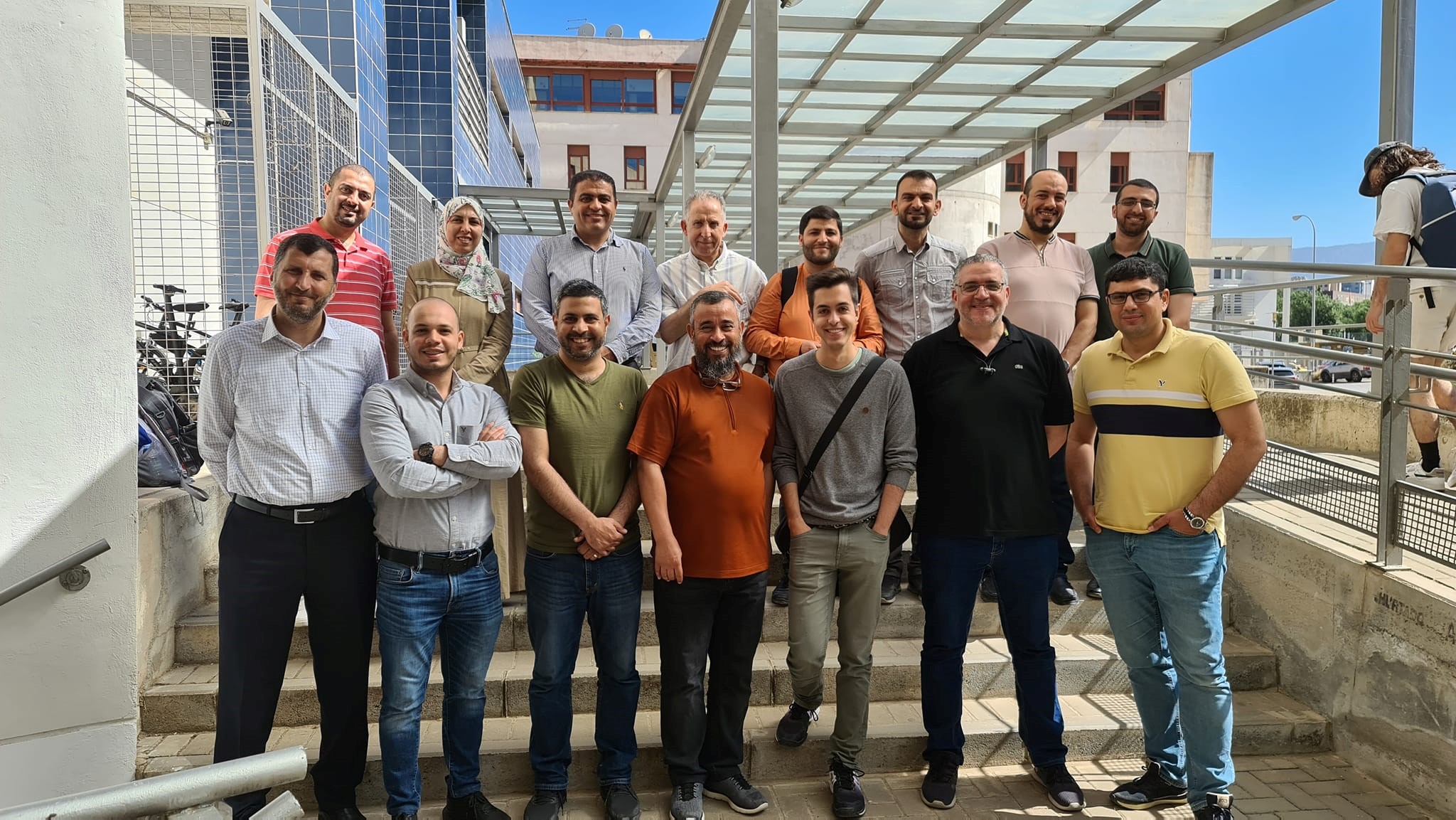
For more information, please contact Professor Abandah at abandah@ju.edu.jo or visit the research group site at https://research.ju.edu.jo/research/groups/MLALP.
References
- G. Abandah, F. Jamour, and E. Qaralleh, “Recognizing handwritten Arabic words using grapheme segmentation and recurrent neural networks," Int'l Journal on Document Analysis and Recognition (IJDAR), Springer, Vol. 17, No. 3, Sep 2014, pp. 275-291, https://doi.org/10.1007/s10032-014-0218-7.
- G. Abandah, A. Graves, B. Al-Shagoor, A. Arabiyat, F. Jamour, and M. Al-Taee, “Automatic diacritization of Arabic text using recurrent neural networks," Int'l Journal on Document Analysis and Recognition (IJDAR), Springer, Vol. 18, No. 2, Jun 2015, pp. 183-197, https://doi.org/10.1007/s10032-015-0242-2.
- G. Abandah, M. Khedher, M. Abdel-Majeed, H. Mansour, S. Hulliel, L. Bisharat, “Classifying and diacritizing Arabic poems using deep recurrent neural networks", Journal of King Saud University - Computer and Information Sciences, Vol. 34, No. 6, Part B, https://doi.org/10.1016/j.jksuci.2020.12.002, June 2022, pp. 3775-3788.
- G. Abandah, A. Suyyagh, M. Abdel-Majeed, “Transfer learning and multi-phase training for accurate diacritization of Arabic poetry", Journal of King Saud University - Computer and Information Sciences, Vol. 34, No. 6, Part B, https://doi.org/10.1016/j.jksuci.2022.04.005, June 2022, pp. 3744-3757.
G. Abandah, A. Suyyagh, M. Khedher, “Correcting Arabic soft spelling mistakes using BiLSTM-based machine learning", International Journal of Advanced Computer Science and Applications (IJACSA), Vol. 13, No. 5, May 2022, pp. 815-829.
http://dx.doi.org/10.14569/IJACSA.2022.0130594.